Does peer-reviewed theory help predict the cross-section of stock returns? (2023)
Andrew Y. Chen, Alejandro Lopez-Lira, Tom Zimmermann
Working Paper, URL
This week’s AGNOSTIC Paper examines the holy grail of empirical research and systematic investing. Is all the research from those smart academics and practitioners really helpful to predict stock returns? Or are we all victims of data mining? The paper if of course not the first one examining this issue, but the approach is in my opinion quite interesting and the authors derive some thought-provoking implications. Pure data mining matches the results from decades of peer-reviewed research surprisingly well. The practical implications, however, are in my opinion not as clear as the statistical ones.
Everything that follows is only my summary of the original paper. So unless indicated otherwise, all tables and charts belong to the authors of the paper and I am just quoting them. The authors deserve full credit for creating this material, so please always cite the original source.
Setup and Idea
The unsolvable core problem of finance, as in many other social sciences, are people. Unlike physics or chemistry, we don’t have the luxury of constant natural laws and the possibility to create virtually infinite trials of controlled experiments. Security prices are what people are willing to buy and sell securities for. As we probably all agree on, people don’t operate under constant laws and are fairly difficult to predict. For those reasons, it is very hard to derive real causal effects in financial markets and we always live with a small probability that our identified significant patterns are nothing but random noise.
Take for example factor investing. There is plenty of empirical and logical evidence that there are some robust patterns and variables that reliably explain security prices. Yet, we can never proof a factor premium like mathematicians can proof their theories. All we can do is minimize the probability that our findings are the result of data mining, i.e. supposedly profitable patterns that are in fact just random coincidences.
All of this is obviously not new and and the debate about data mining in systematic investing goes on since many years. At least in my interpretation, however, academics and practitioners established some best-practices existing to prevent such problems.1I give my best to follow them in this blog. You know what I am talking about: out-of-sample tests, different measures for the same thing, … One of them is theory. The idea is that you don‘t torture the data until you find something interesting (or until you see what you want to see), but start with a clear hypothesis based on economic logic. While this sounds intuitively right, this week‘s authors show that it doesn’t seem as helpful as most of us probably expected. In fact, the authors start with quite provoking statements in the introduction…
In other words, theory helps predict returns if it provides information about expected returns. Our empirical results imply, unfortunately, that theory does not provide such information.
[…]
It’s as if the finance academics are just mining accounting data for return predictability, and then decorating the results with stories about risk and psychology.
Chen et al. (2023, p.2-3)
Data and Methodology
The authors build their work on some popular previous papers that examine the collective effort of academics and practitioners in the factor investing space.2Some of the most prominent are McLean & Pontiff (2016), Jacobs & Müller (2020), Chen & Zimmermann (2022), and Jensen et al. (2023). The core idea and challenge always remains the same. Through the collective efforts of many decentralized researchers and practitioners, the collection of supposedly profitable return predictors ballooned to a situation that it is now known as the factor zoo.3See Cochrance (2011) who, metaphorically, opened the factor zoo… While there is a consensus that most of this work are just different variations of a few more general themes – no serious quant investor I know really believes there are 200+ independently profitable factors – it is very easy to get lost. All of those fancy variables look necessary to predict stock returns and of course, no one publishes a non-profitable backtest.
In theory, all of this sounds pretty obvious and logical. At least for me, however, it is difficult to put a considerable fraction of my wealth in a collection of strategies that simply rank stocks by a few variables. Theory, or at least a plausible idea why a strategy should work and who is on the losing side of the transaction, seems to help here. In one of the key methodological contributions of the paper, the authors develop an interesting approach to test this idea.
They start their analysis with their own Chen & Zimmermann (2022) Open Source Asset Pricing dataset that replicates 200+ return predictors from peer-reviewed academic research.4To the best of my knowledge, this dataset only covers factors in the US equity market. There are some other papers that examine out-of-sample decays internationally, but this is not the focus here. These are both fundamental and technical variables that, according to peer-reviewed articles, significantly predict(ed) future stock returns.
In the next step, they manually classify those predictors into three categories depending on the explanations from the original publications. Risk predictors are strategies where excess returns, as the name suggests, compensate for bearing some kind of risk. For example, some people argue that the long-run returns of the value factor are a compensation for higher bankruptcy risk that comes with cheap crappy companies. Mispricing predictors, in contrast, are strategies that exploit non-rational behavior. A classic example for that is the disposition effect, the tendency of investors to sell winners too early and hold losers for too long, which is essentially the other side of momentum. Finally, they also include the third category Agnostic for predictors where the origins of excess returns are less clear. This is important as most strategies have plausible explanations from both Risk and Mispricing.5This is one aspect of the much greater debate whether financial markets are efficient or not. See this article for an overview. For some more objective robustness, the authors also use natural language processing to calculate the ratio between Risk and Mispricing words. The following table summarizes the results.
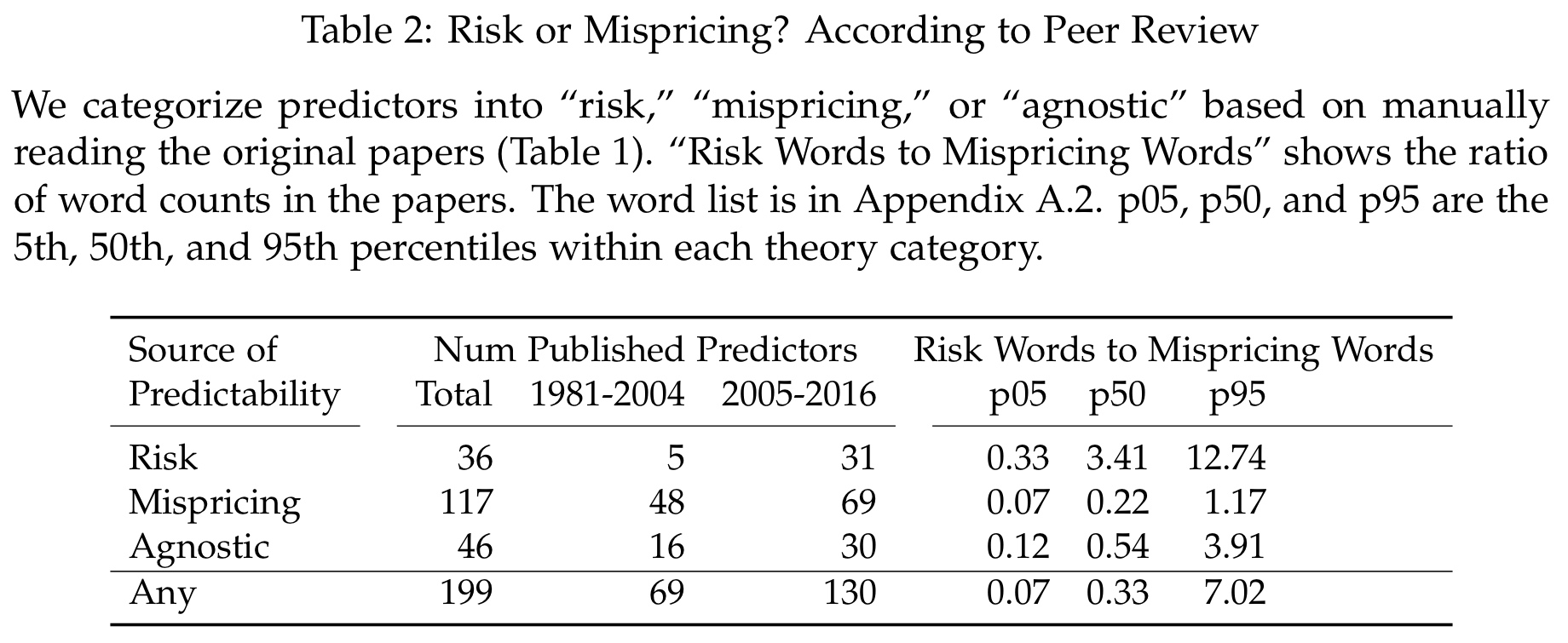
Why are those categories important? Well, the idea is that a sound theory that explains excess returns by some kind of compensated risk is consistent with efficient markets. In contrast, returns that arise from errors of other investors should be much more short-lived. I think this is intuitive. If you get compensated for a risk that doesn’t disappear over time, you can expect to earn those returns as long as the risk exists. Pretty much like an insurance company. If you profit from someone else’s error, however, you must count on the fact that those people don’t learn from their mistakes over time. While this is not necessarily unrealistic if we look at the history of human behavior, it is a much stronger requirement than the risk compensation. The logical conclusion and hypothesis of the authors is therefore that Risk return predictors should be more persistent out-of-sample.
Important Results and Takeaways
Return predictors decay out-of-sample – with and without theory
The following chart summarizes the first core result of the paper. The authors show average monthly long-short returns of return predictors by category in event time. Time t = 0 marks the end of the in-sample publication period, so everything on the right of the y-axis are out-of-sample returns. All strategies are scaled to a monthly average return of 100bps during the in-sample period to isolate the out-of-sample decay.
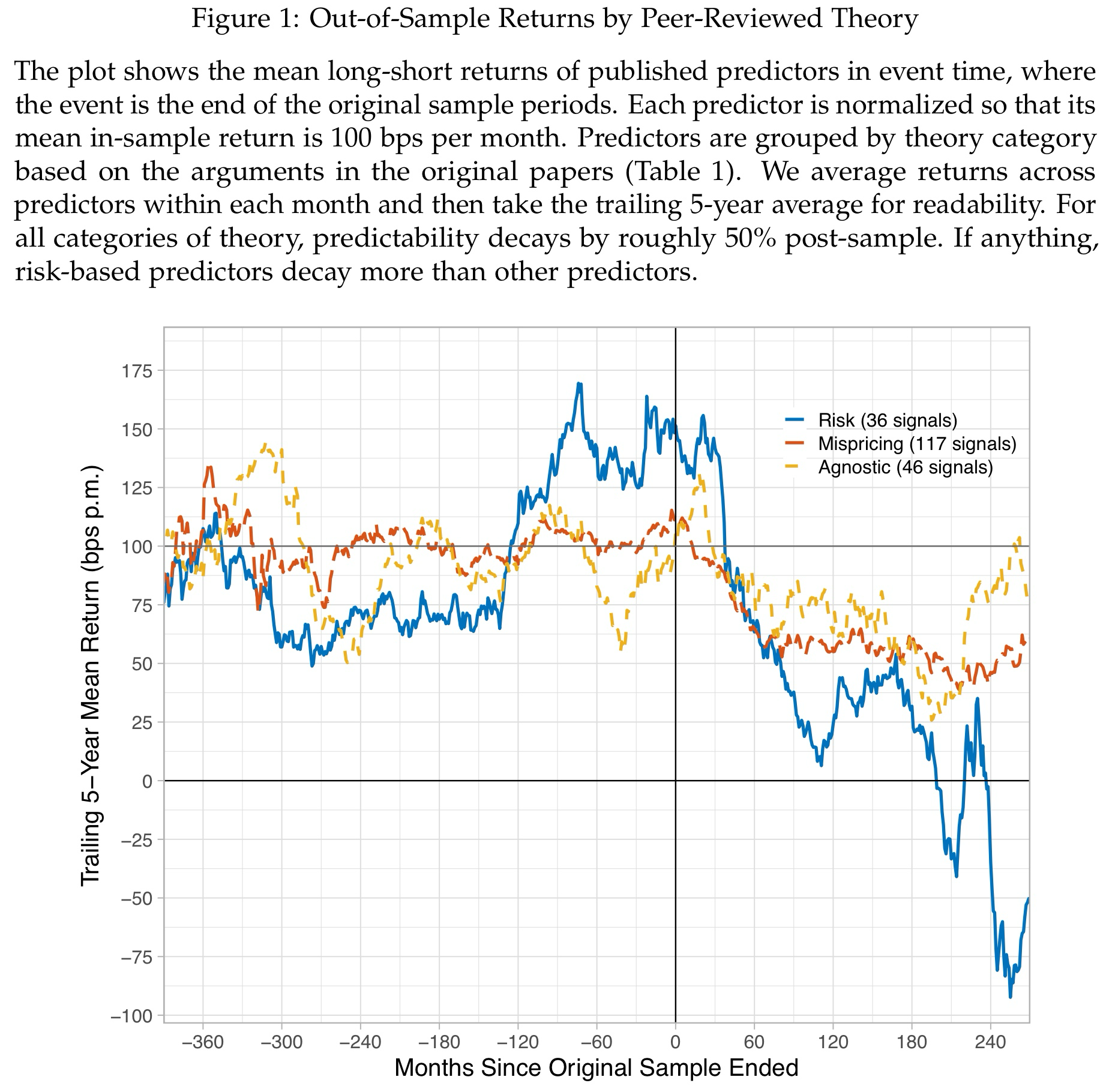
In my opinion, there are two important takeaways. First, there are meaningful out-of-sample decays for virtually all return predictors, no matter the explanation. While the authors interpret this is as strong argument against the whole literature on factor explanations, I think this is just in-line with reasonable expectations. If you have a profitable strategy that others can replicate, you better keep it secret for as long as possible to extract the profits for yourself. Once academics publish papers about it, more people will try to exploit it and it is just reasonable that some of the profits disappear. In my (and many others’) opinion, an out-of-sample decay itself is therefore not necessarily a sign for bad research or data mining. It just reminds us that markets are adaptive systems and you need to constantly innovate to stay ahead of the curve.
Second, and this is more interesting, Risk predictors decay much stronger than their Mispricing and Agnostic counterparts. This is indeed striking as we would expect that excess returns which compensate for some kind of risk should be more persistent than those counting on errors from others (see discussion above). At least based on this analysis, this doesn’t seem to be the case. The authors therefore question whether theory is indeed helpful or even rquired for empirical research and the design of systematic investment strategies.
Data mining generates similar patterns like peer-reviewed research
In the next step, the authors formulate an even more comprehensive attack on the literature and examine if any kind of peer-reviewed research – no matter if Risk, Mispricing, or Agnostic – is worth the effort. For that purpose, they create 29,315 purely data-mined return predictors from interactions of 242 fundamental variables and market equity. Basically, they just rank stocks by all kinds of variables and compute monthly long-short returns. Subsequently, they match the data mined predictors that are closest with respect to monthly average returns and t-statistics to each of the 199 peer-reviewed return predictors.
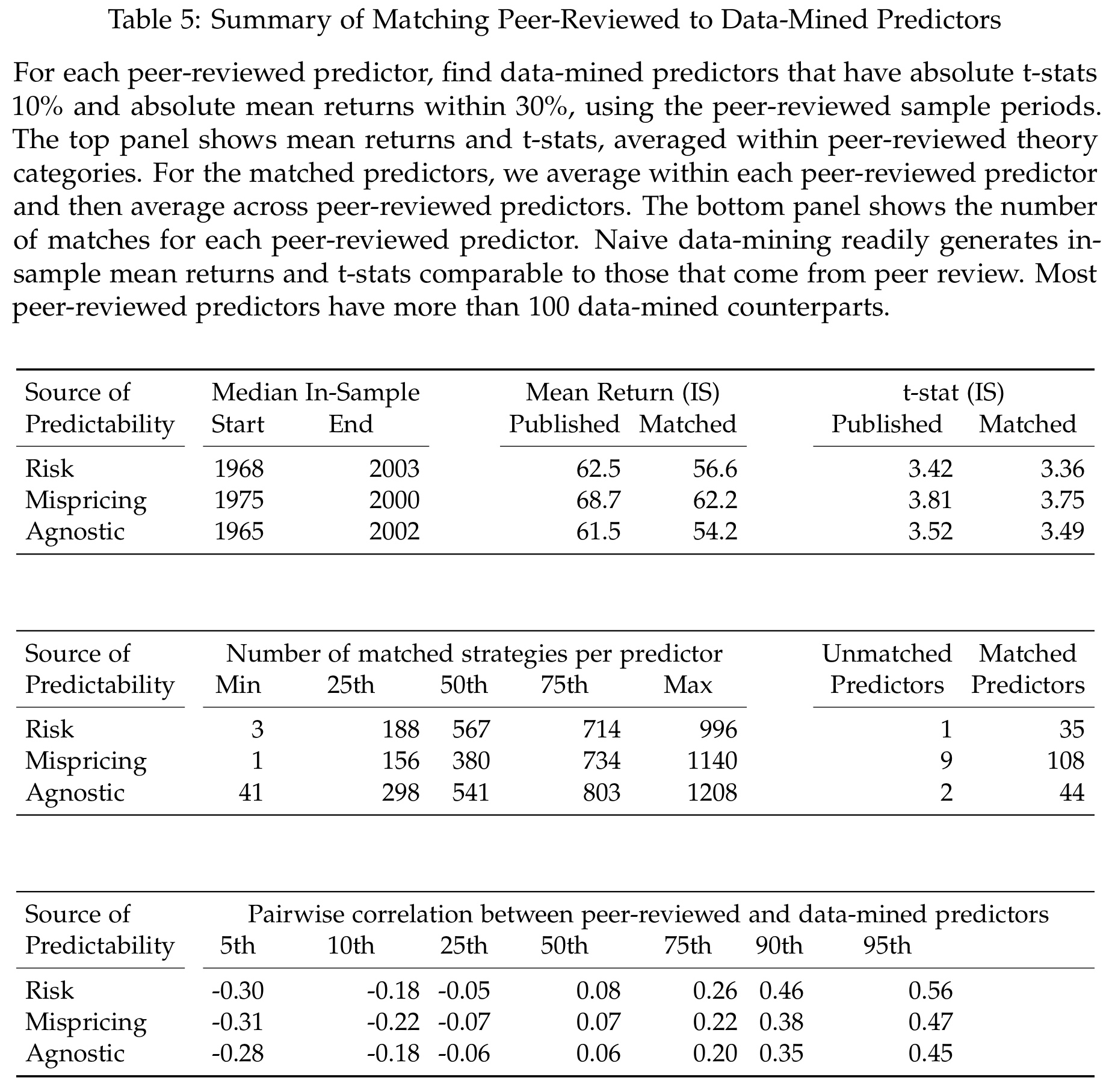
The table above illustrates the result of this procedure in more detail. Of course, it is no surprise that pure data mining can resemble the results from decades of research quite closely. That is just a feature of a social science and messy environment like finance. The precision, however, is astonishing. The t-statistics almost fit the literature to the second decimal. Aside from everything that follows, those results are of course a strong reminder how easy we can fool ourselves with data mining when doing empirical research and how important robust processes are.
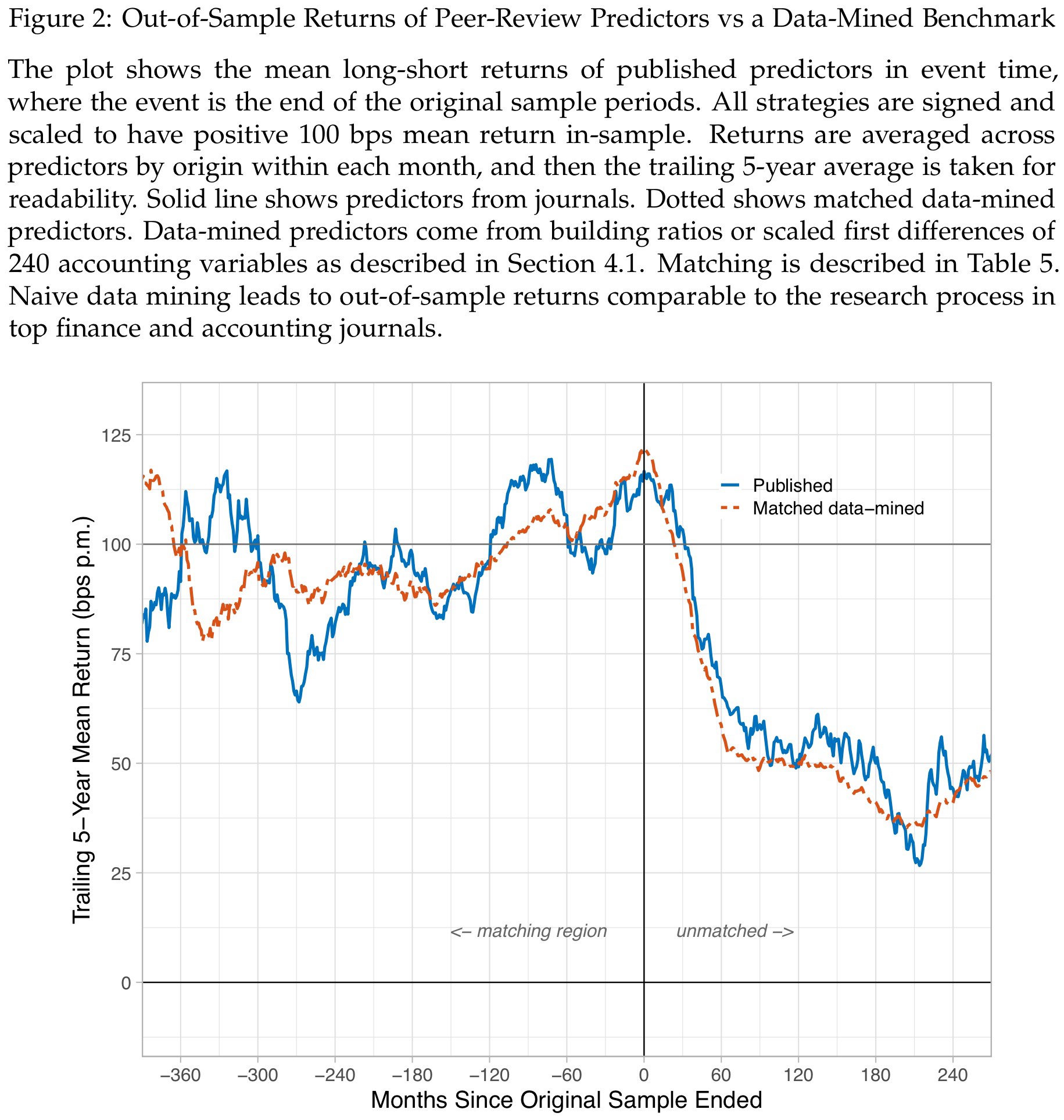
The out-of-sample performance of the data mined strategy are also striking. The chart above shows only minor differences between the performance of peer-reviewed return predictors (blue line) and the data mining matches (red line). The authors therefore, quite provoking, argue that “These results imply that data mining works just as well as reading peer-reviewed journals. Back-testing accounting signals, unguided by theory, leads to the same out-of-sample returns as drawing on the best ideas from the best finance departments in the world.”
The (extremely) theoretical conclusion from those results is simple. Forget about all the efforts regarding robustness, understanding the other side of the trade, having a logical idea why your strategy should work, and just implement a diversified mix of data-mined strategies.6Or do neither of the two, as both approaches have about 50% out-of-sample decay. I will provide more perspective on such conclusions below, but let‘s stay at the paper for now.
Out-of-sample decays are similar for data mining and peer-reviewed research
For even more details, the authors also provide the previous chart separately for each category in the following figure.
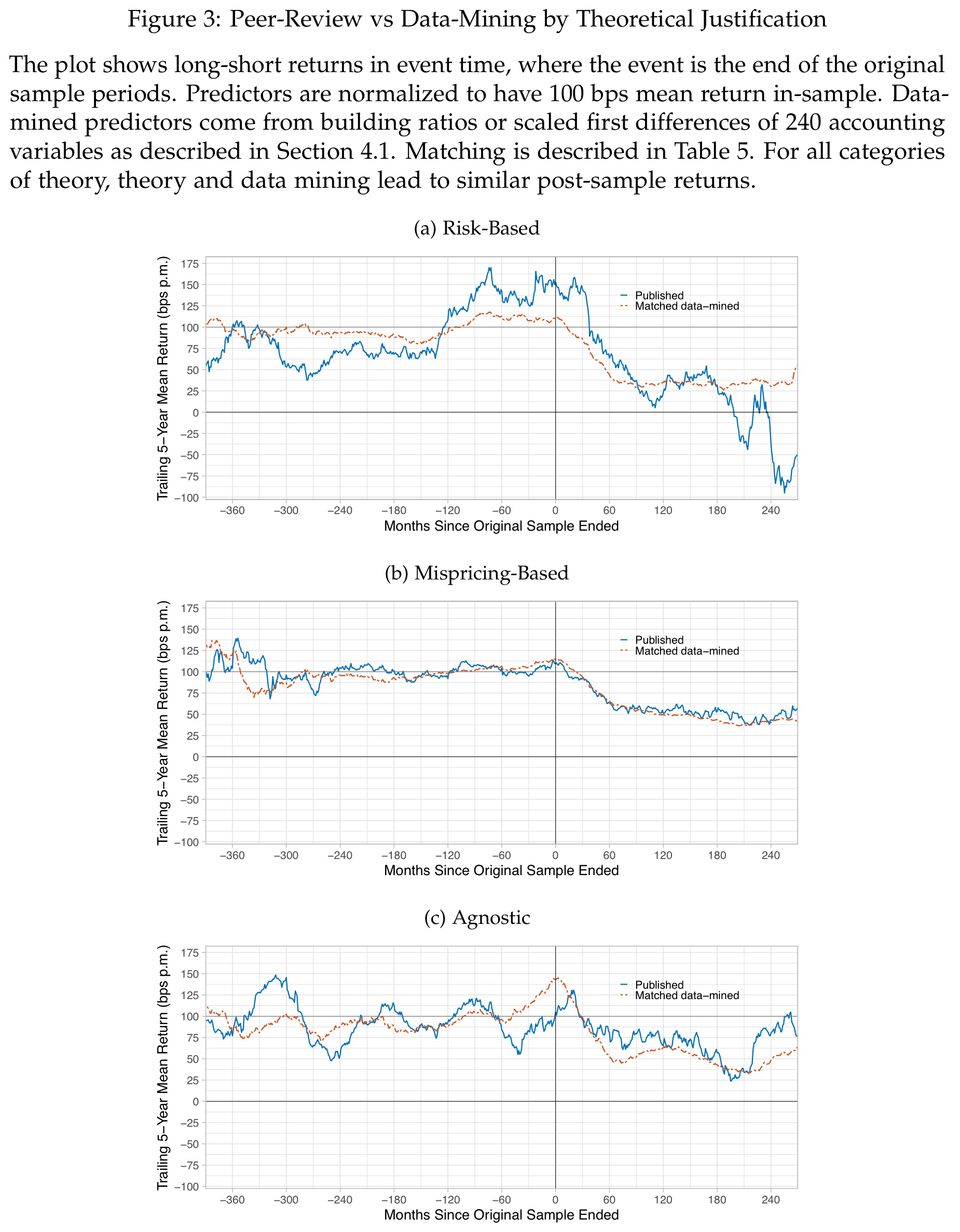
The patterns are of course very similar and again most striking for the supposedly more stable Risk predictors. For each type of return predictor, the data-mined match generates a very similar or sometimes more favorable line than peer-reviewed research. Interestingly, the Agnostic and Mispricing categories exhibit less out-of-sample decay and generally more stable patterns than the Risk predictors. For the reasons mentioned before, this challenges efficient markets as Mispricing seems to be more persistent than thought. In my view, this is an even more interesting contribution from the paper than the out-of-sample decays.
Conclusions and Further Ideas
In general, I really like the idea and analysis of the paper. I also agree with the authors that it is absolutely necessary to examine the history and state of research on factor investing from a broader perspective. Myself being a combination of fundamental value and quantitative factor investing, I always felt uncomfortable with supposedly profitable trading strategies based on one or a few variables.
To understand what I mean, put yourself in the shoes of an investing beginner who consults the literature. I think you wouldn’t draw much value from the collection of 200+ peer-reviewed factors because there is no comprehensive philosophy.7As mentioned earlier, most of the factors are variations of a few general themes. But this doesn’t change the messy state of the literature. Against this background, and the authors mention this explicitly, it seems to be a healthy development that (quantitative) investors now use machine learning to create more comprehensive return forecasts from as many inputs as possible. I think this makes sense because it actually resembles what human portfolio managers do – observe available information and somehow turn them to a portfolio.
Having said that, I still believe there are a few important points to put the insights of the paper in a more practical context.
I’ll skip the risk vs. mispricing stuff, but I will point out for the 1000th time that 50% of a back test out of sample across many factors is a freaking home run.
Cliff Asness via X
The first one are the out-of-sample decays and the strong implications the authors draw from them. The insight that few strategies are as profitable out-of-sample as in their backtests is anything but new. In fact, it is completely reasonable to expect some decay in performance when more people pursue a strategy and/or it becomes publicly available (see the reaction from Cliff Asness on the paper above).
The authors, however, interpret the out-of-sample decays as sign that empirical research on return predictors is basically not much better than pure data mining. Of course, looking at the negative out-of-sample returns of Risk predictors is alarming. In general, however, an out-of-sample decay itself doesn’t mean the underlying research is bad or useless. Even Warren Buffett once stated that his investment performance became worse with every decade.8Check for example this article for a brief analysis. Out-of-sample decays are a fully reasonable feature of competitive financial markets and should be expected. So I believe Cliff Asness is definitely right that any profitable out-of-sample implementation of a backtest is a considerable success.9Who am I to judge if a person who is doing this for 25 years is right on this? Of course he is…
My second point are the practical realities of investment management. Asset managers are typically fiduciaries who manage the money of other people who don’t know as much about investing as they do (hopefully). At least in my interpretation of fiduciary, you should have a reasonable idea what you are doing and be able to explain it to your clients.10I am fully aware that this interpretation unfortunately doesn‘t apply to all players in the industry… I doubt that “I invest in the average return predictor of 29,315 data-mined strategies.” is a helpful explanation in this respect. What I wanna say with this: even though you and I understand the statistics and insights of the paper, normal people don’t care about it and humans tend to like reasons or stories. I mean what would you say if you ask Boeing why their planes fly and you get the answer, “Well, we can reject the hypothesis that it crashes at a t-statistic of 3, but we really don’t care why”?
The third point goes in a similar direction. Even if you are not a fiduciary for other people’s money, investing can be psychologically challenging. Everybody wants to earn excess returns, so it is just natural that it is difficult to get them. Even if you read and understand all the research on things like the equity risk premium, value, momentum, and quality, it is difficult to live through the inevitable drawdowns. It is easy to look at finished drawdowns in a backtest, but it is very hard to lose more and more money without knowing if and when this situation will end. So at least in my experience, you need to believe in your strategies and this is something completely different than looking at historical t-statistics or whatever other figures. I think a reasonable idea why a strategy works and who is on the other side of the trade should help in this respect, even though the authors’ statistics tell another story.
Of course, this discussion is virtually the same as the question whether we need to understand what machine learning models are doing in more sophisticated analyses. The answer is an unsatisfying it depends. For short-term strategies, pure data-mining is probably fine. For example, if you run a strategy that rebalances every two days, it is fine to let the data speak. The feedback loop is short enough to simply stop it when it is no longer working (i.e. you lose money for a few weeks or so). For long-term strategies, however, this is in my opinion very different. To seriously evaluate returns of factors like value, momentum, or low-risk you need at least 3 or 5-year periods, the longer the better. Depending on where you stand, there are unfortunately not so many non-overlapping 5-year periods in a human life. And at least I would have a problem to invest my long-term portfolio in the average return predictor of 29,315 data-mined strategies just to figure out that it didn’t work for whatever reason 10 years later.
Putting all of this together, the authors may be right that peer-reviewed research and theory are (statistically) not helpful to predict stock returns. It is right and necessary to raise this issue and it will hopefully lead to good changes in the academic and practitioner literature. I do believe, however, that theory and rigor research in the sense of understanding what you are attempting to do is helpful for real-world investing.
- AgPa #76: ESG Myth Debunking
- AgPa #75: Optimal Investment Committees
- AgPa #74: Peer-Reviewed Research is Not Helpful to Predict Returns – Really?
- AgPa #73: Country and Industry Momentum
This content is for educational and informational purposes only and no substitute for professional or financial advice. The use of any information on this website is solely on your own risk and I do not take responsibility or liability for any damages that may occur. The views expressed on this website are solely my own and do not necessarily reflect the views of any organisation I am associated with. Income- or benefit-generating links are marked with a star (*). All content that is not my intellectual property is marked as such. If you own the intellectual property displayed on this website and do not agree with my use of it, please send me an e-mail and I will remedy the situation immediately. Please also read the Disclaimer.
Endnotes
1 | I give my best to follow them in this blog. You know what I am talking about: out-of-sample tests, different measures for the same thing, … |
---|---|
2 | Some of the most prominent are McLean & Pontiff (2016), Jacobs & Müller (2020), Chen & Zimmermann (2022), and Jensen et al. (2023). |
3 | See Cochrance (2011) who, metaphorically, opened the factor zoo… |
4 | To the best of my knowledge, this dataset only covers factors in the US equity market. There are some other papers that examine out-of-sample decays internationally, but this is not the focus here. |
5 | This is one aspect of the much greater debate whether financial markets are efficient or not. See this article for an overview. |
6 | Or do neither of the two, as both approaches have about 50% out-of-sample decay. |
7 | As mentioned earlier, most of the factors are variations of a few general themes. But this doesn’t change the messy state of the literature. |
8 | Check for example this article for a brief analysis. |
9 | Who am I to judge if a person who is doing this for 25 years is right on this? Of course he is… |
10 | I am fully aware that this interpretation unfortunately doesn‘t apply to all players in the industry… |